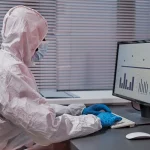
Seven Steps to Implement a Data Cleaning Process
2 December 2024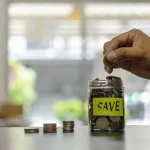
Seven Benefits From Data Warehouse Reports
14 December 2024Integrating Machine Learning with Your EDW for Enhanced Decision-Making
The Role of EDWs and ML in Modern Organisations
An EDW is a centralised repository for data aggregation, storage, and retrieval. It provides a unified view of your organisation's data, enabling seamless reporting and analysis. However, traditional EDWs often focus on historical data analysis, limiting their potential for predictive insights.
Machine learning, on the other hand, uses algorithms to identify patterns and make predictions based on data. By integrating ML into your EDW, you can move beyond static reporting to dynamic and predictive analytics, unlocking new opportunities for growth and efficiency.
Benefits of Integrating ML with Your EDW
- Enhanced Predictive Analytics: ML algorithms can analyse historical data in your EDW to forecast future trends, such as customer behaviour, market changes, or supply chain demands.
- Improved Operational Efficiency: Automating repetitive tasks, such as anomaly detection or fraud identification, reduces manual effort and enhances accuracy.
- Personalised Customer Experiences: By leveraging ML, you can segment customers and predict preferences, enabling tailored marketing strategies and improved customer satisfaction.
- Real-Time Decision Making: Combining real-time data ingestion with ML models can provide immediate insights, crucial for time-sensitive decisions.
Steps to Integrate ML with Your EDW
- Assess Your Data Architecture
Evaluate the current state of your EDW to ensure it can support ML integration. Key considerations include scalability, data accessibility, and processing power.
- Clean and Prepare Your Data
Machine learning models require high-quality data. Use data cleaning techniques to remove duplicates, fill missing values, and standardise formats.
- Choose the Right ML Tools
Select tools and frameworks that align with your organisation's goals. Popular options include TensorFlow, PyTorch, and Azure Machine Learning.
- Integrate ML Models into Your Workflow
Develop and integrate ML models with your EDW using APIs or ETL (extract, transform, load) pipelines. It enables seamless data flow and real-time model updates.
- Monitor and Optimise
Continuously monitor the performance of your ML models and refine them to ensure they adapt to changing data patterns and business needs.
Challenges to Anticipate
- Data Silos: Fragmented data sources can hinder integration. Invest in data unification strategies to overcome this challenge.
- Skill Gaps: Implementing ML requires expertise in data science and engineering. Consider upskilling your team or hiring specialists.
- Cost Considerations: Building and maintaining ML systems can be expensive. Optimise costs by leveraging cloud-based ML platforms.
Real-World Examples
- Retail Industry: A global retailer integrated ML with their EDW to forecast demand and optimise inventory levels, reducing stockouts and overstocking.
- Healthcare: Hospitals use ML-powered EDWs to predict patient admission rates and allocate resources effectively.
- Finance: Financial institutions leverage ML models for fraud detection and credit risk assessment, enhancing security and decision-making.
Conclusion
Integrating machine learning with your EDW is not just a technological upgrade; it's a strategic shift that can empower your organisation to thrive in a competitive environment. By harnessing the power of ML, you can transform your data into a dynamic asset, driving innovation and delivering measurable business value. Embrace this integration to stay ahead of the curve and unlock the full potential of your data.